Detecting Arctic charr spawning nests with a drone
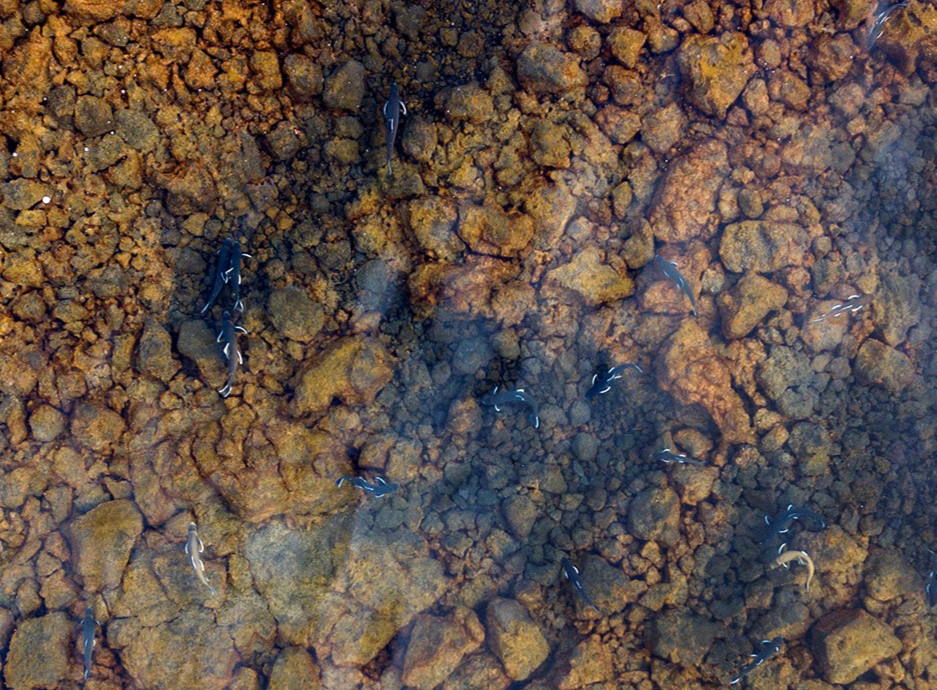
Today I get to talk about my own paper!
Last year we published an article where we introduced a method we developed to detect and monitor Salmonid spawning grounds using a drone and machine learning. Spawning nests are where the fish release their eggs and the eggs develop until the fish hatch. You can see what the nests look like in the image below. Before spawning (when they release their eggs) female fish clean the rocks on the lake bottom from sand and algae. This makes the rocks stand out due to their dark colouring.
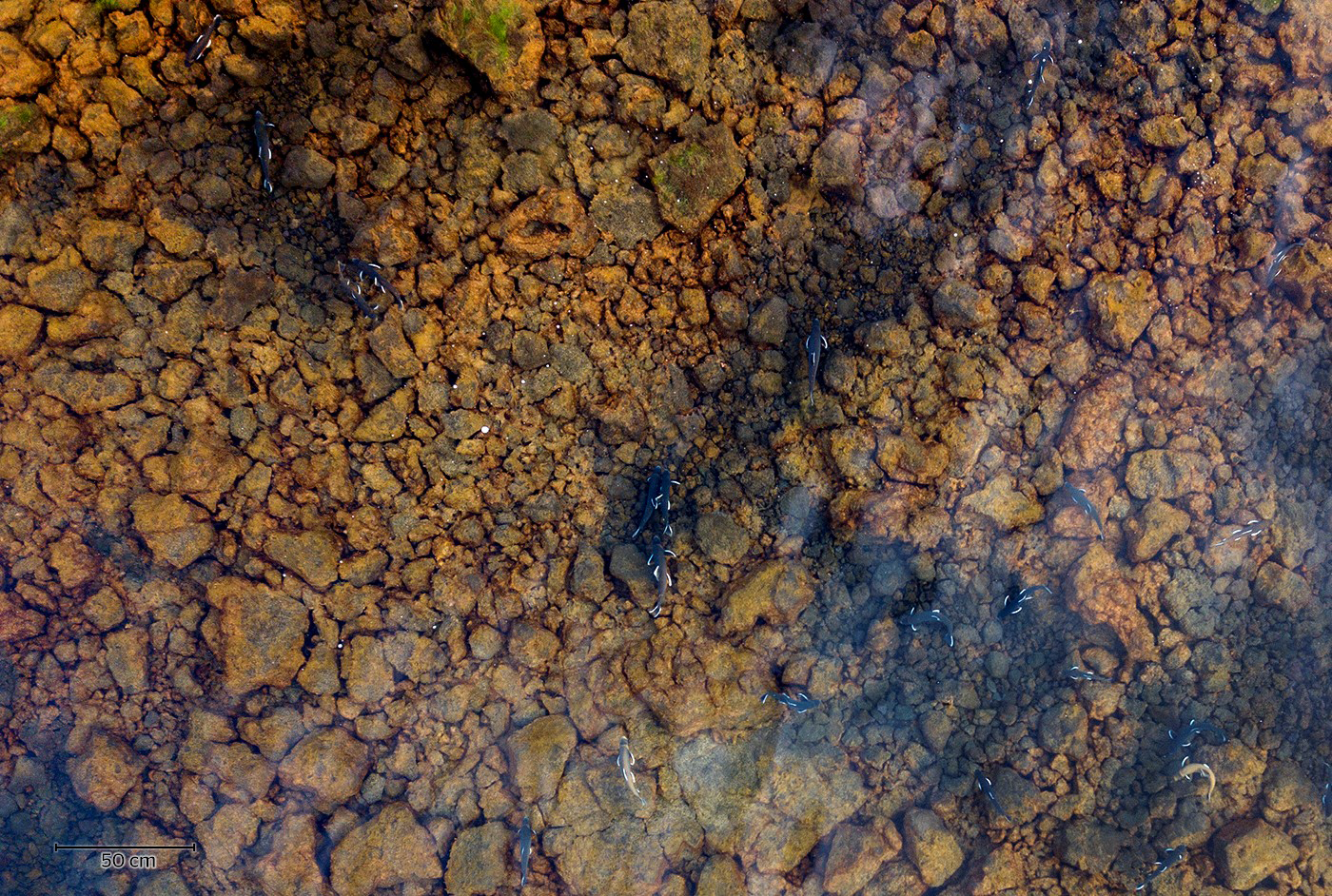
Why is this so important?
Salmonids (a family of fish that includes salmon “lax”, Arctic charr “bleikja”, and trout “urriði”) are very vulnerable during their early development when they are growing in the spawning grounds. So monitoring of these grounds is important for the future of these fish. However, their monitoring is not done often and when it is done, it is often done by manually counting the nests. This can result in under- and overestimation of the counting and will give incorrect information.
Luckily, spawning grounds are visible from above (as you can see in the image above) and a drone can thus be used to map them. So during this project, we developed a method that can map spawning grounds.
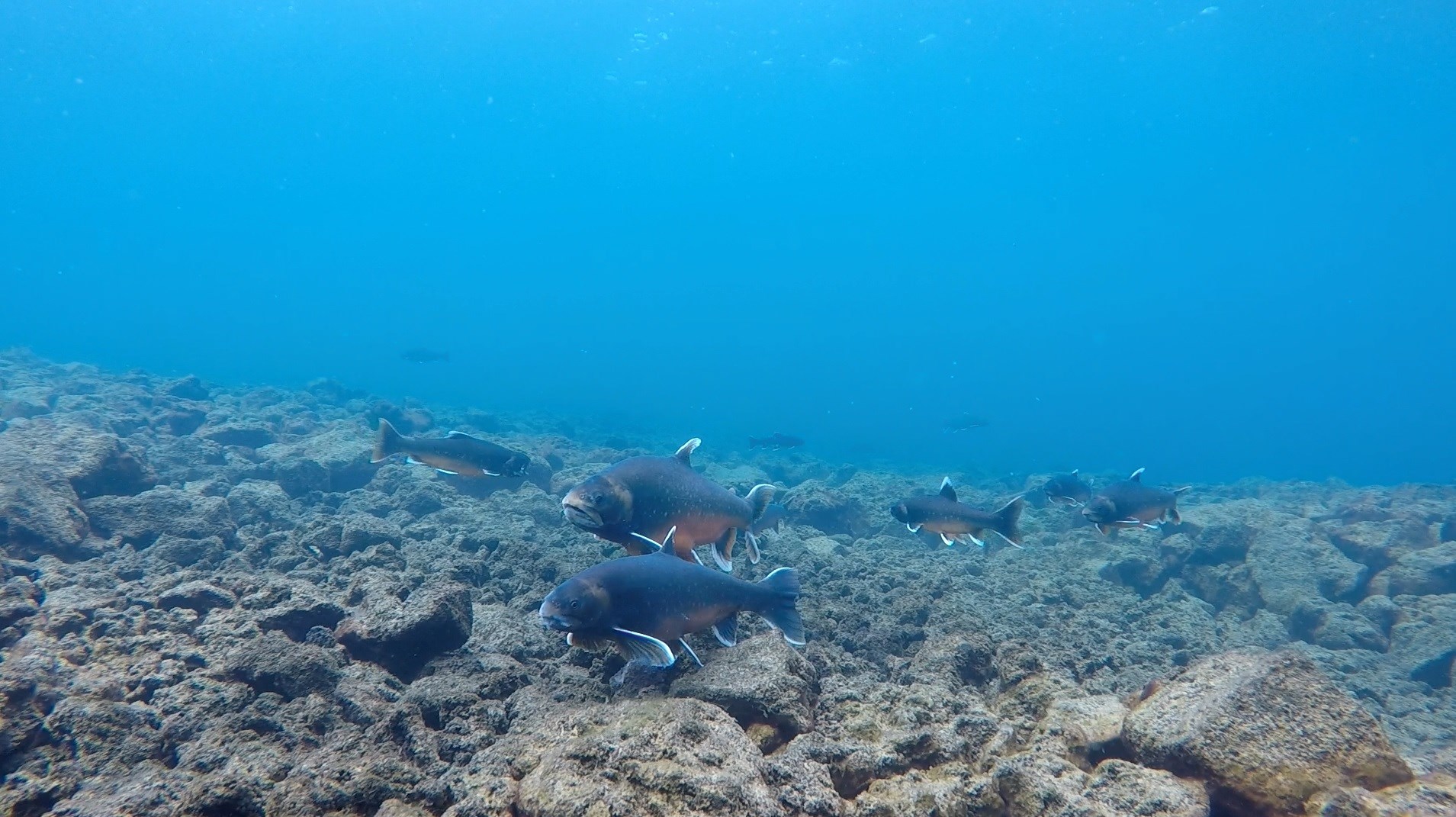
Flying a drone and training the algorithm
The first step of this method is to fly the drone where you know the spawning grounds are located. We chose the spawning grounds of Arctic charr (“bleikja”) in Thingvallavatn as we know a lot of information about these grounds already and the nests are highly visible. We checked different drone heights for taking the images and found that flying the drone at 50 meters was the best to detect the spawning grounds. After taking an image of the spawning grounds it is time to train your machine learning algorithm. An image is made up of pixels and we selected 1000 pixels for each feature we wanted to classify. We classified spawning nests, underwater rocks, vegetation, deep water, shoreline and surface rocks (because those are the features we saw in our image). After selecting these 1000 pixels for each feature the algorithm takes care of the rest and classifies the whole image for you as you can see in the figure below.
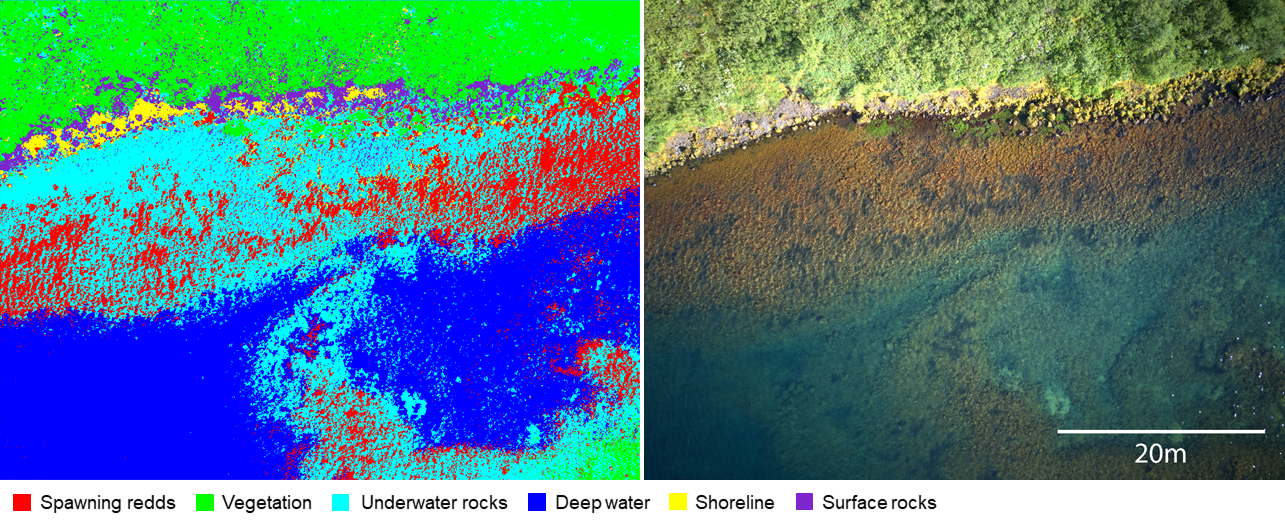
We also checked whether our method was accurate and we found that all features were classified with high accuracies. Most importantly, the accuracy of detecting spawning nests was >90%.
Everyone can be a scientist
What we are most enthusiastic about is that this is quite a simple method to use. Anyone with a drone can simply fly it at a 50-meter height when the weather is good and take images. This allows for crowdsourcing where images can be taken by the general public and sent in for analysis. This makes frequent monitoring of spawning grounds so much easier as we are often limited by time and manpower. Also, this method can be applied to other lakes and rivers, and other salmonid species. So there is a lot of potential for this method to be used on a large scale.
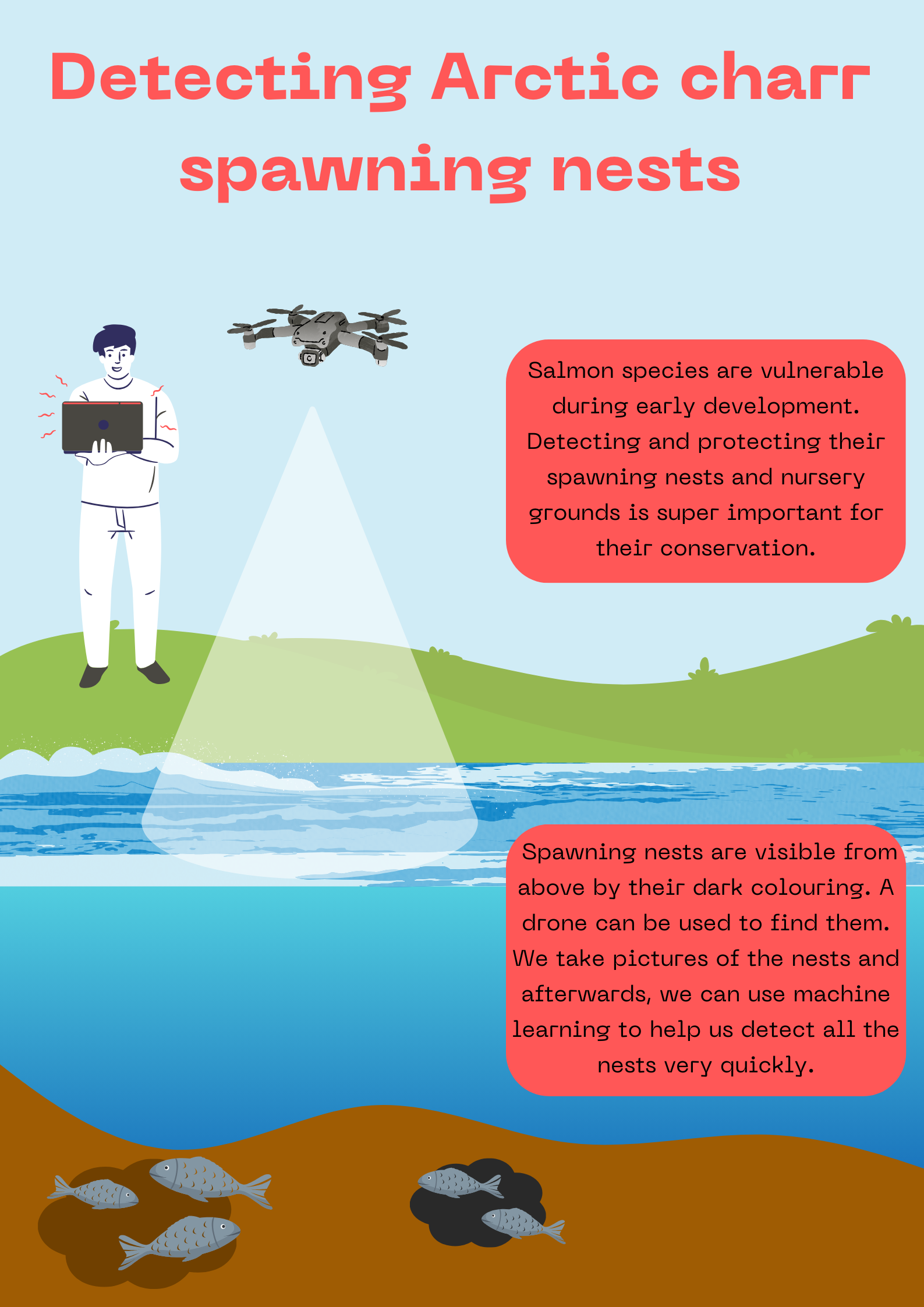